class: front .pull-left-wide[ # Estadística Correlacional] .pull-right-narrow[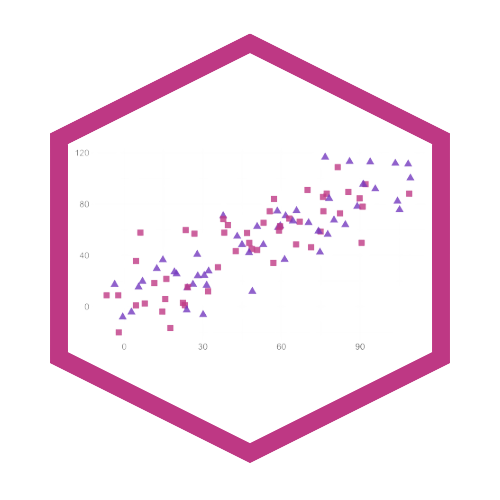] ## Asociación, inferencia y reporte ---- .pull-left[ ## Juan Carlos Castillo ## Sociología FACSO - UChile ## 2do Sem 2023 ## [.orange[correlacional.netlify.com]](https:/correlacional.netlify.com) ] .pull-right-narrow[ .center[ .content-block-gray[ ## Sesión 4: ## .orange[Tamaños de efecto, otros coeficientes y matrices]] ] ] --- layout: true class: animated, fadeIn --- class: roja ## Objetivos de la sesión de hoy ### 1. Calcular e interpretar el coeficiente de determinación ### 2. Aprender a interpretar los tamaños de efecto del coeficiente de correlación ### 3. Conocer, calcular e interpretar coeficientes de correlación alternativos para datos ordinales ### 4. Reportar e interpretar matrices de correlación --- class: middle center # Lectura: Field 205-244 Correlation --- class: roja right # .black[Contenidos] .pull-left-narrow[ ] .pull-right-wide[ ### .yellow[1- Resumen sesión anterior] ### 2- Magnitud de la correlación ### 3- Otros coeficientes de correlación ### 4- Matrices de correlación ] --- ## Hacia la covarianza .pull-left[ 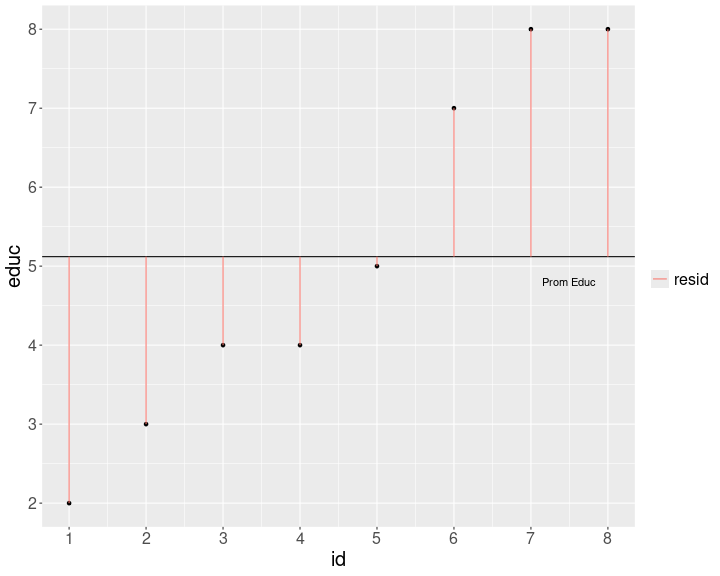<!-- --> .center[ ### Educación ] ] .pull-right[ 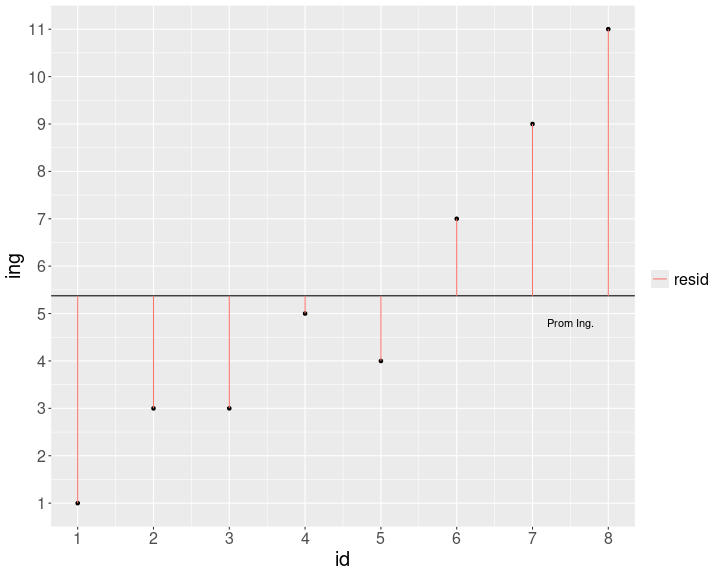<!-- --> .center[ ### Ingreso ] ] --- # Covarianza .pull-left[ .center[ ### Varianza educación (x) `$$\sigma_{edu}^{2}={\sum_{i=1}^{N}(x_{i}-\bar{x})^{2}\over {N - 1}}$$` `$$\sigma_{edu}^{2}={\sum_{i=1}^{N}(x_{i}-\bar{x})(x_{i}-\bar{x})\over {N - 1}}$$` ] ] .pull-right[ .center[ ### Varianza ingreso (y) `$$\sigma_{ing}^{2}={\sum_{i=1}^{N}(y_{i}-\bar{y})^{2}\over {N - 1}}$$` `$$\sigma_{ing}^{2}={\sum_{i=1}^{N}(y_{i}-\bar{y})(y_{i}-\bar{y})\over {N - 1}}$$` ] ] -- .content-box-red[ `$$Covarianza=cov(x,y) = \frac{\sum_{i=1}^{N}(x_i - \bar{x})(y_i - \bar{y})} {N-1}$$` ] --- ## Cálculo correlación .pull-left[ .small[ <div style="border: 1px solid #ddd; padding: 0px; overflow-y: scroll; height:450px; overflow-x: scroll; width:500px; "><table> <thead> <tr> <th style="text-align:right;position: sticky; top:0; background-color: #FFFFFF;"> educ </th> <th style="text-align:right;position: sticky; top:0; background-color: #FFFFFF;"> ing </th> <th style="text-align:right;position: sticky; top:0; background-color: #FFFFFF;"> dif_m_educ2 </th> <th style="text-align:right;position: sticky; top:0; background-color: #FFFFFF;"> dif_m_ing2 </th> <th style="text-align:right;position: sticky; top:0; background-color: #FFFFFF;"> dif_xy </th> </tr> </thead> <tbody> <tr> <td style="text-align:right;"> 2 </td> <td style="text-align:right;"> 1 </td> <td style="text-align:right;"> 9.77 </td> <td style="text-align:right;"> 19.14 </td> <td style="text-align:right;"> 13.67 </td> </tr> <tr> <td style="text-align:right;"> 3 </td> <td style="text-align:right;"> 3 </td> <td style="text-align:right;"> 4.52 </td> <td style="text-align:right;"> 5.64 </td> <td style="text-align:right;"> 5.05 </td> </tr> <tr> <td style="text-align:right;"> 4 </td> <td style="text-align:right;"> 3 </td> <td style="text-align:right;"> 1.27 </td> <td style="text-align:right;"> 5.64 </td> <td style="text-align:right;"> 2.67 </td> </tr> <tr> <td style="text-align:right;"> 4 </td> <td style="text-align:right;"> 5 </td> <td style="text-align:right;"> 1.27 </td> <td style="text-align:right;"> 0.14 </td> <td style="text-align:right;"> 0.42 </td> </tr> <tr> <td style="text-align:right;"> 5 </td> <td style="text-align:right;"> 4 </td> <td style="text-align:right;"> 0.02 </td> <td style="text-align:right;"> 1.89 </td> <td style="text-align:right;"> 0.17 </td> </tr> <tr> <td style="text-align:right;"> 7 </td> <td style="text-align:right;"> 7 </td> <td style="text-align:right;"> 3.52 </td> <td style="text-align:right;"> 2.64 </td> <td style="text-align:right;"> 3.05 </td> </tr> <tr> <td style="text-align:right;"> 8 </td> <td style="text-align:right;"> 9 </td> <td style="text-align:right;"> 8.27 </td> <td style="text-align:right;"> 13.14 </td> <td style="text-align:right;"> 10.42 </td> </tr> <tr> <td style="text-align:right;"> 8 </td> <td style="text-align:right;"> 11 </td> <td style="text-align:right;"> 8.27 </td> <td style="text-align:right;"> 31.64 </td> <td style="text-align:right;"> 16.17 </td> </tr> </tbody> </table></div> ] ] .pull-right[ `$$r=\frac{\sum(x-\bar{x})(y-\bar{y})}{\sqrt{\sum(x-\bar{x})^{2} \sum(y-\bar{y})^{2}}}$$` ``` r sum(data$dif_xy); sum(data$dif_m_educ2);sum(data$dif_m_ing2) ``` ``` [1] 51.625 ``` ``` [1] 36.875 ``` ``` [1] 79.875 ``` ] --- ## Cálculo correlación .pull-left[ `\begin{align*} r &= \frac{\sum(x-\bar{x})(y-\bar{y})}{\sqrt{\sum(x-\bar{x})^{2} \sum(y-\bar{y})^{2}}} \\ \\ &= \frac{51.625}{ \sqrt{36.875*79.875}} \\ \\ &= \frac{51.625}{54.271} \\ \\ &= 0.951 \end{align*}` ] -- .pull-right[ ``` r cor(data$educ,data$ing) ``` ``` [1] 0.9512367 ``` ] --- # Nubes de puntos (scatterplot) .center[ 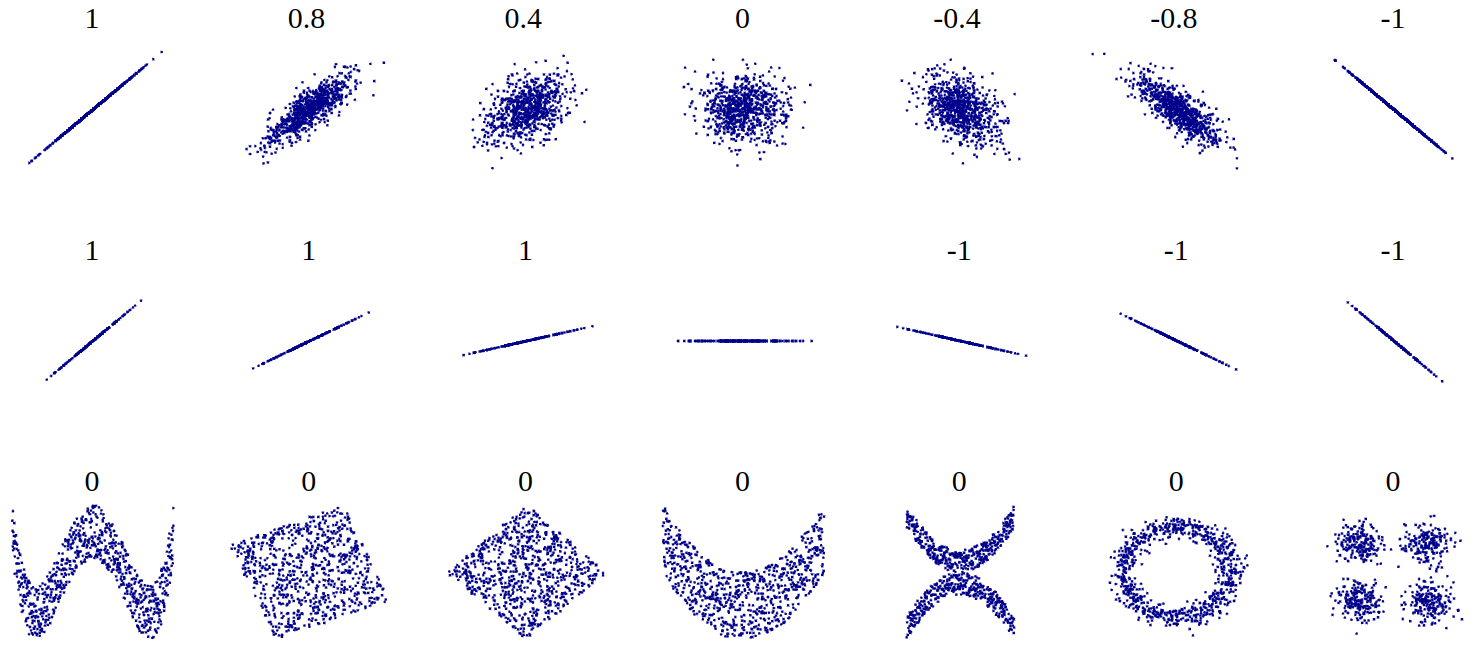 ] --- class: roja ## Interpretación - El coeficiente de correlación (de Pearson) es una medida de asociación lineal entre variables, que indica el sentido y la fuerza de la asociación -- - Varía entre +1 y -1, donde - valores .black[positivos] indican relación directa (aumenta una, aumenta la otra) - valores .black[negativos] indican relación inversa (aumenta una, disminuye la otra) --- class: roja right # .black[Contenidos] .pull-left-narrow[ ] .pull-right-wide[ ### 1- Resumen sesión anterior ### .yellow[2- Magnitud de la correlación] ### 3- Otros coeficientes de correlación ### 4- Matrices de correlación ] --- .pull-left[ Si dos variables covarían, entonces .red[comparten varianza]  ] -- .pull-right[ <br> <br> # .red[¿Cuánta varianza comparten? ] ] --- # Estableciendo la varianza compartida .pull-left[ La varianza compartida puede pensarse como 1 - la varianza no compartida (o varianza única) La varianza no compartida se asocia al concepto de .red[residuos], es decir, la cantidad de varianza que no está contenida en la correlación ] .pull-right[ 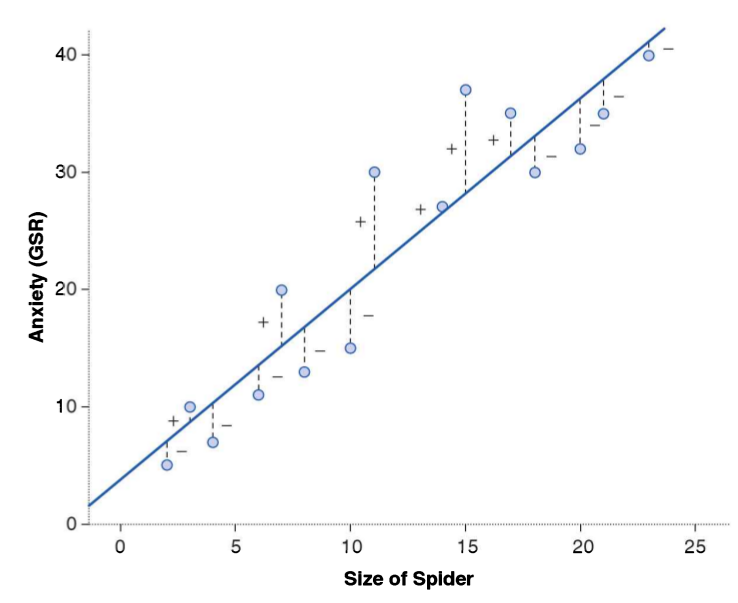 ] --- # Estableciendo la varianza compartida .pull-left[ Para poder obtener los residuos vamos a generar una .red[recta] que represente la asociación entre las variables. Esta es la recta de **regresión** <sup>1</sup> Esta recta nos permite obtener un valor estimado de `\(y\)` para cada valor de `\(x\)` <br> <br> <br> <br> <br> <br> <br> <br> <br> .small[[1] Detalles próximo semestre] ] .pull-right[ 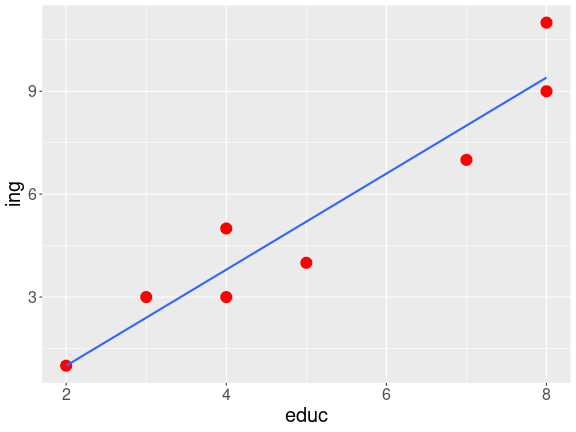<!-- --> ] --- .pull-left[ .small[ ``` r reg1 <- lm(ing ~ educ, data=data) data$predict <-predict.lm(reg1) plot2 <-ggplot(data, aes(x=educ, y=predict)) + geom_point( colour = "red", size = 5) + theme(text = element_text(size = 20))+ stat_smooth(method = "lm", se = FALSE, fullrange = T) ``` ] ] .pull-right[ .small[ ``` r plot2 ``` 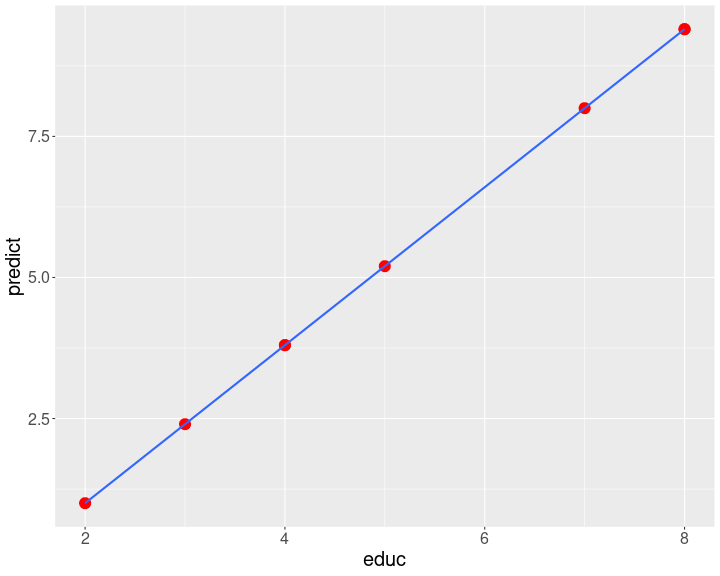<!-- --> ] ] --- .center[  ] `\begin{align*} SS_{tot}&=SS_{reg} + SS_{error} \\ \Sigma(y_i - \bar{y})^2&=\Sigma (\hat{y}_i-\bar{y})^2 +\Sigma(y_i-\hat{y}_i)^2 \end{align*}` --- # Varianza compartida `$$SS_{tot}=SS_{reg} + SS_{error}$$` -- `$$\frac{SS_{tot}}{SS_{tot}}=\frac{SS_{reg}}{SS_{tot}} + \frac{SS_{error}}{SS_{tot}}$$` -- `$$1=\frac{SS_{reg}}{SS_{tot}}+\frac{SS_{error}}{SS_{tot}}$$` `$$\frac{SS_{reg}}{SS_{tot}}= 1- \frac{SS_{error}}{SS_{tot}}=R^2$$` --- # ¿Qué relación tienen `\(R^2\)` y `\(r\)` (correlación)? .pull-left[ `\(R^2=r^2\)` Por lo tanto, en nuestro ejemplo: .small[ ``` r cor(data$educ,data$ing) ``` ``` [1] 0.9512367 ``` ] `\begin{align*} r&= 0.95 \\ r^2&=0.95 ^2 \\ r^2&=0.902 = R^2 \end{align*}` ] -- .pull-right[ .content-box-red[ Intepretación: _El porcentaje de varianza compartida entre educación e ingreso es de 90%._ _Es decir, ambas variables comparten el 90% de su varianza_ ]] --- # `\(R^2\)` o coeficiente de determinación - ¿Cuánto de los ingresos se asocia a educación, y viceversa? -- - el `\(R^2\)` - es la proporción de la varianza de Y que se asocia a X - varía entre 0 y 1, y usualmente se expresa en porcentaje --- # Criterios de Cohen para tamaños de efecto - El coeficiente de correlación `\(r\)` de Pearson nos indica la dirección y la fuerza/intensidad de la asociación. - Pero, ¿qué nos dice el tamaño del coeficiente? Por ejemplo, si el coeficiente es 0.5, ¿esto es pequeño, mediano o grande? - Cohen (1988, 1992) sugiere una serie de criterios convencionales para clasificar efectos como pequeños, medianos o grandes. --- class: center  --- class: middle ## Para el caso de correlación de Pearson, Cohen sugiere: - tamaño de efecto .roja[pequeño]: alrededor de .red[0.10] - tamaño de efecto .roja[mediano]: alrededor de .red[0.30] - tamaño de efecto .roja[grande]: alrededor de .red[0.50] y más --- # Resumen - **Coeficiente de determinación** `\(R^2\)`: varianza compartida entre variables - **Tamaño de efecto:** valores convencionales para establecer si una magnitud es pequeña, mediana o grande. - Varianza compartida y tamaño de efecto son relevantes de reportar junto a la información de inferencia y significación estadística (próxima unidad) --- class: roja right # .black[Contenidos] .pull-left-narrow[ ] .pull-right-wide[ ### 1- Resumen sesión anterior ### 2- Magnitud de la correlación ### .yellow[3- Otros coeficientes de correlación] ### 4- Matrices de correlación ] --- # Coeficiente de correlación de Spearman - se utiliza para variables ordinales y/o cuando se se violan supuestos de distribución normal - es equivalente a la correlación de Pearson del ranking de las observaciones analizadas - es alta cuando las observaciones tienen un ranking similar --- # Cálculo Spearman - se le asigna un número de ranking a cada valor - el valor más bajo obtiene el mayor ranking, y el más alto el menor - en caso de valores repetidos se produce un "empate", y entonces el ranking se promedia. --- # Ejemplo: variable Educación .medium[ .pull-left-narrow[ ``` r data$educ ``` ``` [1] 2 3 4 4 5 7 8 8 ``` Como estos valores están ordenados de menor a mayor, entonces en principio los valores de ranking serían: `8 7 6 5 4 3 2 1`. ] .pull-right-wide[ <br> <br> Pero, hay un par de empates - el valor 4 está repetido y corresponden a los ranking 6 y 5, por lo tanto a ambos se les asigna el promedio de estos rankings: 5,5 - lo mismo sucede con el valor 8 en los rankings 2 y 1, por lo tanto a ambos se les asigna el valor 1,5 ] ] --- count: false .panel1-spearman-auto[ ``` r *data ``` ] .panel2-spearman-auto[ ``` id educ ing mean_educ dif_m_educ dif_m_educ2 mean_ing dif_m_ing dif_m_ing2 1 1 2 1 5.125 -3.125 9.765625 5.375 -4.375 19.140625 2 2 3 3 5.125 -2.125 4.515625 5.375 -2.375 5.640625 3 3 4 3 5.125 -1.125 1.265625 5.375 -2.375 5.640625 4 4 4 5 5.125 -1.125 1.265625 5.375 -0.375 0.140625 5 5 5 4 5.125 -0.125 0.015625 5.375 -1.375 1.890625 6 6 7 7 5.125 1.875 3.515625 5.375 1.625 2.640625 7 7 8 9 5.125 2.875 8.265625 5.375 3.625 13.140625 8 8 8 11 5.125 2.875 8.265625 5.375 5.625 31.640625 dif_xy predict 1 13.671875 1.0 2 5.046875 2.4 3 2.671875 3.8 4 0.421875 3.8 5 0.171875 5.2 6 3.046875 8.0 7 10.421875 9.4 8 16.171875 9.4 ``` ] --- count: false .panel1-spearman-auto[ ``` r data %>% * select (educ, ing) ``` ] .panel2-spearman-auto[ ``` educ ing 1 2 1 2 3 3 3 4 3 4 4 5 5 5 4 6 7 7 7 8 9 8 8 11 ``` ] --- count: false .panel1-spearman-auto[ ``` r data %>% select (educ, ing) %>% * mutate(., educ_rank=c(8,7,5.5,5.5,4,3,1.5,1.5)) ``` ] .panel2-spearman-auto[ ``` educ ing educ_rank 1 2 1 8.0 2 3 3 7.0 3 4 3 5.5 4 4 5 5.5 5 5 4 4.0 6 7 7 3.0 7 8 9 1.5 8 8 11 1.5 ``` ] --- count: false .panel1-spearman-auto[ ``` r data %>% select (educ, ing) %>% mutate(., educ_rank=c(8,7,5.5,5.5,4,3,1.5,1.5)) %>% * mutate(., ing_rank=c(8,6.5,6.5,4,5,3,2,1)) ``` ] .panel2-spearman-auto[ ``` educ ing educ_rank ing_rank 1 2 1 8.0 8.0 2 3 3 7.0 6.5 3 4 3 5.5 6.5 4 4 5 5.5 4.0 5 5 4 4.0 5.0 6 7 7 3.0 3.0 7 8 9 1.5 2.0 8 8 11 1.5 1.0 ``` ] --- count: false .panel1-spearman-auto[ ``` r data %>% select (educ, ing) %>% mutate(., educ_rank=c(8,7,5.5,5.5,4,3,1.5,1.5)) %>% mutate(., ing_rank=c(8,6.5,6.5,4,5,3,2,1)) *cor(data_spr$educ_rank, data_spr$ing_rank) ``` ] .panel2-spearman-auto[ ``` educ ing educ_rank ing_rank 1 2 1 8.0 8.0 2 3 3 7.0 6.5 3 4 3 5.5 6.5 4 4 5 5.5 4.0 5 5 4 4.0 5.0 6 7 7 3.0 3.0 7 8 9 1.5 2.0 8 8 11 1.5 1.0 ``` ``` [1] 0.9394112 ``` ] <style> .panel1-spearman-auto { color: black; width: 38.6060606060606%; hight: 32%; float: left; padding-left: 1%; font-size: 80% } .panel2-spearman-auto { color: black; width: 59.3939393939394%; hight: 32%; float: left; padding-left: 1%; font-size: 80% } .panel3-spearman-auto { color: black; width: NA%; hight: 33%; float: left; padding-left: 1%; font-size: 80% } </style> --- Cálculo directo en `R`: ``` r cor.test(data$educ, data$ing, "two.sided", "spearman") ``` ``` Spearman's rank correlation rho data: data$educ and data$ing S = 5.0895, p-value = 0.0005311 alternative hypothesis: true rho is not equal to 0 sample estimates: rho 0.9394112 ``` --- # Coeficiente de correlación Tau de Kendall .pull-left[ - Recomendado cuando hay un set de datos pequeños y/o cuando hay mucha repetición de observaciones en el mismo ranking - Se basa en una comparación de pares de observaciones concordantes y discordantes ] -- .pull-right[ En `R`: .small[ ``` r cor.test(data$educ, data$ing, "two.sided", "kendall") ``` ``` Kendall's rank correlation tau data: data$educ and data$ing z = 2.9115, p-value = 0.003597 alternative hypothesis: true tau is not equal to 0 sample estimates: tau 0.8680791 ``` ] ] --- class: inverse ## Recomendaciones generales - Pearson es el coeficiente de correlación por defecto - En caso de datos en escala de medición ordinal se puede aplicar Spearman (aunque Pearson es también aceptado en este contexto). - Kendall se reporta en casos muy específicos donde hay un set de datos pequeños y repetición de observaciones en el mismo ranking ("empates") --- class: roja right # .black[Contenidos] .pull-left-narrow[ ] .pull-right-wide[ ### 1- Resumen sesión anterior ### 2- Magnitud de la correlación ### 3- Otros coeficientes de correlación ### .yellow[4- Matrices de correlación] ] --- # Matriz de correlación - una matriz de correlación se conforma cuando se representa simultaneamente más de un par de asociaciones bivariadas - por ejemplo, si agregamos la variable edad a nuestra base de datos: ``` r data$edad <- c(50, 65, 27, 15, 40, 22, 25, 38) ``` Tenemos .red[3 variables], y por lo tanto los siguentes pares de correlaciones posibles: ingreso-educación, ingreso-edad, y educación-edad --- count: false .panel1-matriz1-auto[ ``` r *cor_mat <- data ``` ] .panel2-matriz1-auto[ ] --- count: false .panel1-matriz1-auto[ ``` r cor_mat <- data %>% * select(educ, ing, edad) ``` ] .panel2-matriz1-auto[ ] --- count: false .panel1-matriz1-auto[ ``` r cor_mat <- data %>% select(educ, ing, edad) %>% * cor(.) ``` ] .panel2-matriz1-auto[ ] --- count: false .panel1-matriz1-auto[ ``` r cor_mat <- data %>% select(educ, ing, edad) %>% cor(.) *cor_mat ``` ] .panel2-matriz1-auto[ ``` educ ing edad educ 1.0000000 0.9512367 -0.4704649 ing 0.9512367 1.0000000 -0.4058455 edad -0.4704649 -0.4058455 1.0000000 ``` ] --- count: false .panel1-matriz1-auto[ ``` r cor_mat <- data %>% select(educ, ing, edad) %>% cor(.) cor_mat *round(cor_mat, 3) ``` ] .panel2-matriz1-auto[ ``` educ ing edad educ 1.0000000 0.9512367 -0.4704649 ing 0.9512367 1.0000000 -0.4058455 edad -0.4704649 -0.4058455 1.0000000 ``` ``` educ ing edad educ 1.000 0.951 -0.470 ing 0.951 1.000 -0.406 edad -0.470 -0.406 1.000 ``` ] --- count: false .panel1-matriz1-auto[ ``` r cor_mat <- data %>% select(educ, ing, edad) %>% cor(.) cor_mat round(cor_mat, 3) *sjPlot::tab_corr(cor_mat) ``` ] .panel2-matriz1-auto[ ``` educ ing edad educ 1.0000000 0.9512367 -0.4704649 ing 0.9512367 1.0000000 -0.4058455 edad -0.4704649 -0.4058455 1.0000000 ``` ``` educ ing edad educ 1.000 0.951 -0.470 ing 0.951 1.000 -0.406 edad -0.470 -0.406 1.000 ``` <table style="border-collapse:collapse; border:none;"> <tr> <th style="font-style:italic; font-weight:normal; border-top:double black; border-bottom:1px solid black; padding:0.2cm;"> </th> <th style="font-style:italic; font-weight:normal; border-top:double black; border-bottom:1px solid black; padding:0.2cm;">educ</th> <th style="font-style:italic; font-weight:normal; border-top:double black; border-bottom:1px solid black; padding:0.2cm;">ing</th> <th style="font-style:italic; font-weight:normal; border-top:double black; border-bottom:1px solid black; padding:0.2cm;">edad</th> </tr> <tr> <td style="font-style:italic;">educ</td> <td style="padding:0.2cm; text-align:center;"> </td> <td style="padding:0.2cm; text-align:center;">0.951</td> <td style="padding:0.2cm; text-align:center;">-0.470</td> </tr> <tr> <td style="font-style:italic;">ing</td> <td style="padding:0.2cm; text-align:center;">0.951</td> <td style="padding:0.2cm; text-align:center;"> </td> <td style="padding:0.2cm; text-align:center;">-0.406</td> </tr> <tr> <td style="font-style:italic;">edad</td> <td style="padding:0.2cm; text-align:center;">-0.470</td> <td style="padding:0.2cm; text-align:center;">-0.406</td> <td style="padding:0.2cm; text-align:center;"> </td> </tr> <tr> <td colspan="4" style="border-bottom:double black; border-top:1px solid black; font-style:italic; font-size:0.9em; text-align:right;">Computed correlation used pearson-method with listwise-deletion.</td> </tr> </table> ] <style> .panel1-matriz1-auto { color: black; width: 38.6060606060606%; hight: 32%; float: left; padding-left: 1%; font-size: 80% } .panel2-matriz1-auto { color: black; width: 59.3939393939394%; hight: 32%; float: left; padding-left: 1%; font-size: 80% } .panel3-matriz1-auto { color: black; width: NA%; hight: 33%; float: left; padding-left: 1%; font-size: 80% } </style> --- # Matriz de correlaciones - tabla de doble entrada donde las variables se presentan tanto en las filas como en las columnas - el coeficiente de correlación correspondiente al par de variables aparece en la intersección de las columnas - existe información redundante - las correlaciones se repiten, dado que las variables se intersectan dos veces en la tabla - la diagonal tiene solo unos (1), ya que es la correlación de la variable consigo misma --- count: false # Ajustando tabla sjPlot::tab_corr .panel1-matriz2-auto[ ``` r *sjPlot::tab_corr(cor_mat, * triangle = "lower", * title = "Tabla de correlaciones del ejemplo" * ) ``` ] .panel2-matriz2-auto[ <table style="border-collapse:collapse; border:none;"> <caption style="font-weight: bold; text-align:left;">Tabla de correlaciones del ejemplo</caption> <tr> <th style="font-style:italic; font-weight:normal; border-top:double black; border-bottom:1px solid black; padding:0.2cm;"> </th> <th style="font-style:italic; font-weight:normal; border-top:double black; border-bottom:1px solid black; padding:0.2cm;">educ</th> <th style="font-style:italic; font-weight:normal; border-top:double black; border-bottom:1px solid black; padding:0.2cm;">ing</th> <th style="font-style:italic; font-weight:normal; border-top:double black; border-bottom:1px solid black; padding:0.2cm;">edad</th> </tr> <tr> <td style="font-style:italic;">educ</td> <td style="padding:0.2cm; text-align:center;"> </td> <td style="padding:0.2cm; text-align:center;"> </td> <td style="padding:0.2cm; text-align:center;"> </td> </tr> <tr> <td style="font-style:italic;">ing</td> <td style="padding:0.2cm; text-align:center;">0.951</td> <td style="padding:0.2cm; text-align:center;"> </td> <td style="padding:0.2cm; text-align:center;"> </td> </tr> <tr> <td style="font-style:italic;">edad</td> <td style="padding:0.2cm; text-align:center;">-0.470</td> <td style="padding:0.2cm; text-align:center;">-0.406</td> <td style="padding:0.2cm; text-align:center;"> </td> </tr> <tr> <td colspan="4" style="border-bottom:double black; border-top:1px solid black; font-style:italic; font-size:0.9em; text-align:right;">Computed correlation used pearson-method with listwise-deletion.</td> </tr> </table> ] <style> .panel1-matriz2-auto { color: black; width: 38.6060606060606%; hight: 32%; float: left; padding-left: 1%; font-size: 80% } .panel2-matriz2-auto { color: black; width: 59.3939393939394%; hight: 32%; float: left; padding-left: 1%; font-size: 80% } .panel3-matriz2-auto { color: black; width: NA%; hight: 33%; float: left; padding-left: 1%; font-size: 80% } </style> --- count: false # Matriz con librería corrplot .panel1-matriz3-auto[ ``` r *library(corrplot) ``` ] .panel2-matriz3-auto[ ] --- count: false # Matriz con librería corrplot .panel1-matriz3-auto[ ``` r library(corrplot) *corrplot(cor_mat) ``` ] .panel2-matriz3-auto[ <!-- --> ] <style> .panel1-matriz3-auto { color: black; width: 38.6060606060606%; hight: 32%; float: left; padding-left: 1%; font-size: 80% } .panel2-matriz3-auto { color: black; width: 59.3939393939394%; hight: 32%; float: left; padding-left: 1%; font-size: 80% } .panel3-matriz3-auto { color: black; width: NA%; hight: 33%; float: left; padding-left: 1%; font-size: 80% } </style> --- count: false # Matriz con librería corrplot .panel1-matriz31-auto[ ``` r *corrplot(cor_mat, * method = 'number') ``` ] .panel2-matriz31-auto[ <!-- --> ] <style> .panel1-matriz31-auto { color: black; width: 38.6060606060606%; hight: 32%; float: left; padding-left: 1%; font-size: 80% } .panel2-matriz31-auto { color: black; width: 59.3939393939394%; hight: 32%; float: left; padding-left: 1%; font-size: 80% } .panel3-matriz31-auto { color: black; width: NA%; hight: 33%; float: left; padding-left: 1%; font-size: 80% } </style> --- count: false # corrplot .panel1-matriz4-auto[ ``` r *corrplot(cor_mat, * method = 'number', * type = 'lower', * number.cex = 3, * tl.cex = 3, * diag = FALSE) ``` ] .panel2-matriz4-auto[ <!-- --> ] <style> .panel1-matriz4-auto { color: black; width: 38.6060606060606%; hight: 32%; float: left; padding-left: 1%; font-size: 80% } .panel2-matriz4-auto { color: black; width: 59.3939393939394%; hight: 32%; float: left; padding-left: 1%; font-size: 80% } .panel3-matriz4-auto { color: black; width: NA%; hight: 33%; float: left; padding-left: 1%; font-size: 80% } </style> --- count: false # corrplot.mixed .panel1-matriz5-auto[ ``` r *corrplot.mixed(cor_mat, * lower = "number", * upper = "circle", * number.cex = 3, * tl.cex = 3, * diag = "n") ``` ] .panel2-matriz5-auto[ <!-- --> ] <style> .panel1-matriz5-auto { color: black; width: 38.6060606060606%; hight: 32%; float: left; padding-left: 1%; font-size: 80% } .panel2-matriz5-auto { color: black; width: 59.3939393939394%; hight: 32%; float: left; padding-left: 1%; font-size: 80% } .panel3-matriz5-auto { color: black; width: NA%; hight: 33%; float: left; padding-left: 1%; font-size: 80% } </style> --- count: false # corrplot.mixed .panel1-matriz6-auto[ ``` r *corrplot(cor_mat, * type = "lower", * addCoef.col = 'white', * number.cex = 3, * tl.cex = 3, * diag = FALSE) ``` ] .panel2-matriz6-auto[ <!-- --> ] <style> .panel1-matriz6-auto { color: black; width: 38.6060606060606%; hight: 32%; float: left; padding-left: 1%; font-size: 80% } .panel2-matriz6-auto { color: black; width: 59.3939393939394%; hight: 32%; float: left; padding-left: 1%; font-size: 80% } .panel3-matriz6-auto { color: black; width: NA%; hight: 33%; float: left; padding-left: 1%; font-size: 80% } </style> --- count: false # corrplot.mixed .panel1-matriz7-auto[ ``` r *corrplot(cor_mat, * type = "lower", * addCoef.col = 'white', * number.cex = 3, * tl.cex = 3, * diag = FALSE, * method = 'square') ``` ] .panel2-matriz7-auto[ <!-- --> ] <style> .panel1-matriz7-auto { color: black; width: 38.6060606060606%; hight: 32%; float: left; padding-left: 1%; font-size: 80% } .panel2-matriz7-auto { color: black; width: 59.3939393939394%; hight: 32%; float: left; padding-left: 1%; font-size: 80% } .panel3-matriz7-auto { color: black; width: NA%; hight: 33%; float: left; padding-left: 1%; font-size: 80% } </style> --- # Consideraciones sobre casos perdidos - Cuando hay casos perdidos en las variables, ¿cuál es el número de casos de la matriz de correlaciones? - Las correlaciones bivariadas se calculan con información completa, por lo tanto si hay un dato perdido en una de las variables se elimina el caso completo - Algunas funciones lo hacen de manera automática, en otras hay que especificarlo previamente --- # Ejemplo Agreguemos un caso perdido (NA en R) a una de nuestras variables ``` r data$edad ``` ``` [1] 50 65 27 15 40 22 25 38 ``` ``` r data$edad <-replace(data$edad, data$edad==15, NA) data$edad ``` ``` [1] 50 65 27 NA 40 22 25 38 ``` --- .medium[ ``` r cormat_NA <- data %>% select(educ, ing, edad) %>% cor(.) round(cormat_NA,3) ``` ``` educ ing edad educ 1.000 0.951 NA ing 0.951 1.000 NA edad NA NA 1 ``` ``` r cormat_listwise <- data %>% select(educ, ing, edad) %>% cor(., use = "complete.obs") round(cormat_listwise, 3) ``` ``` educ ing edad educ 1.000 0.962 -0.669 ing 0.962 1.000 -0.494 edad -0.669 -0.494 1.000 ``` ] --- # Eliminación de casos perdidos por lista (o listwise) - las correlaciones bivariadas requieren eliminación de casos perdidos tipo listwise, es decir, si hay un dato perdido en una variable se pierde el caso completo - Para conocer el número de casos con que se calculó la matriz: .medium[ ``` r sum(complete.cases(data)) ``` ``` [1] 7 ``` ] Por lo tanto, en el cálculo se perdió el caso o fila completa de la base (de 8 casos) que tenía el caso perdido. --- # Eliminación de casos perdidos por pares (o pairwise) - en el caso de las matrices de correlaciones es posible tomar la opción .red[pairwise] para casos perdidos - pairwise quiere decir que se eliminan los casos perdidos solo cuando afectan al cálculo de un par específico. - en el caso de nuestro ejemplo, si consideramos listwise todas las correlaciones tienen 7 casos, pero con pairwise la correlación entre educación e ingreso mantendría 8 casos. - por lo tanto, pairwise permite mayor rescate de información y mayor N en el cálculo de matrices de correlaciones --- # Opción pairwise en correlación en R ``` r data %>% select(educ, ing, edad) %>% cor(., use = "pairwise") ``` ``` educ ing edad educ 1.0000000 0.9512367 -0.6693607 ing 0.9512367 1.0000000 -0.4941732 edad -0.6693607 -0.4941732 1.0000000 ``` En este caso vemos una leve variación en el coeficiente comprometido (educ-ing) comparando listwise con pairwise --- # Número de casos pairwise ``` r data %>% select(educ,ing,edad) %>% psych::pairwiseCount() ``` ``` educ ing edad educ 8 8 7 ing 8 8 7 edad 7 7 7 ``` Se indica que en las correlaciones con edad se utilizaron 7 casos, mientras en la correlación entre ingreso y educación se utilizan 8 casos con el método pairwise --- class: inverse ## Resumen .medium[ - Reporte de correlación: además de reportar la intensidad y el sentido, acompañar reporte de tamaño de efecto y varianza compartida `\(R^2\)` - Correlación de Spearman: apropiada para variables ordinales, equivale a la correlación de Pearson del ranking de las variables - Matriz de correlaciones: forma tradicional de reporte de asociaciones de las variables de una investigación, importante considerar tratamiento de datos perdidos (listwise o pairwise) ] --- # ASISTENCIA .pull-left[ 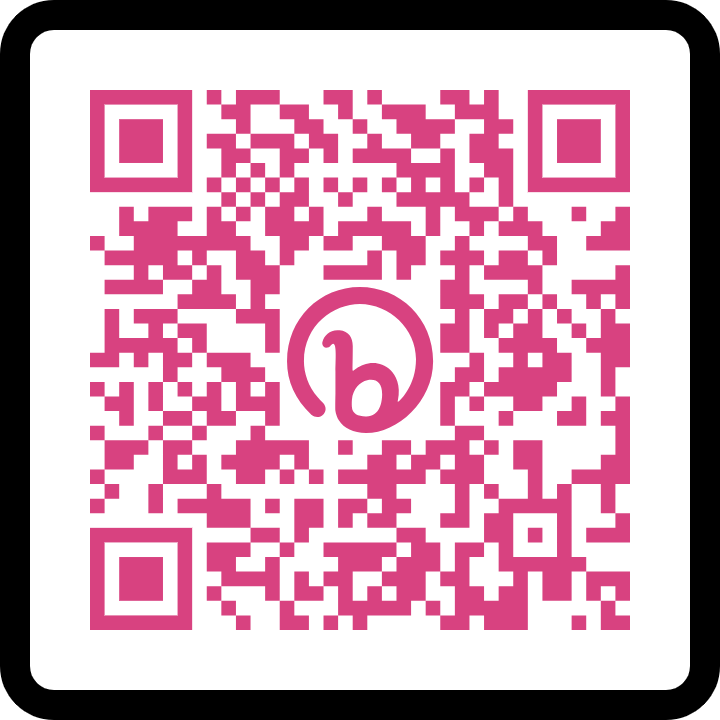 ] .pull-right[ <br> <br> <br> <br> <br> bit.ly/correlacional-asistencia ] --- class: front .pull-left-wide[ # Estadística Correlacional] .pull-right-narrow[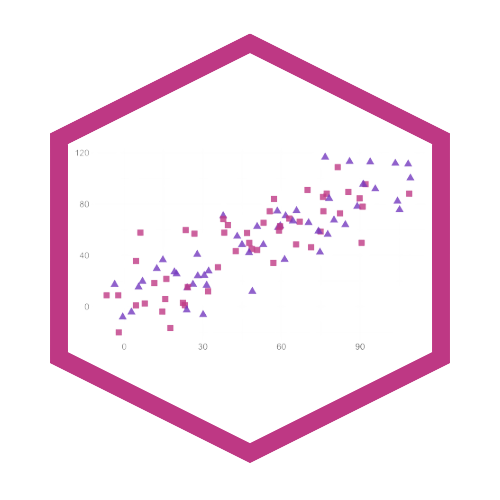] ## Asociación, inferencia y reporte ---- .pull-left[ ## Juan Carlos Castillo ## Sociología FACSO - UChile ## 2do Sem 2023 ## [.orange[correlacional.netlify.com]](https://encuestas-sociales.netlify.com) ] <!-- adjust font size in this css code chunk for flipbook, currently 80 -->